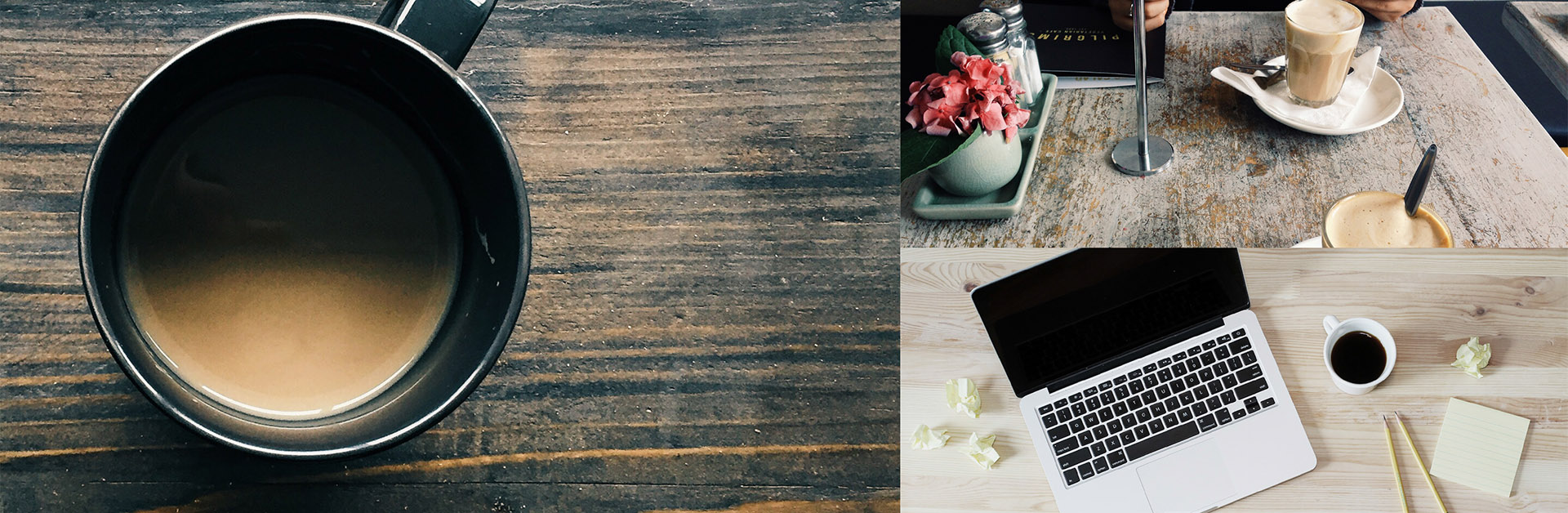
February 21, 2022
B K Pradhan et al, 2022. Automated Detection of Caffeinated Coffee-Induced Short-Term Effects on ECG Signals Using EMD, DWT, and WPD, Nutrients, Volume 14.
ABSTRACT:
The effect of coffee (caffeinated) on electro-cardiac activity is not yet sufficiently researched. In the current study, the occurrence of coffee-induced short-term changes in electrocardiogram (ECG) signals was examined. Further, a machine learning model that can efficiently detect coffee-induced alterations in cardiac activity is proposed. The ECG signals were decomposed using three different joint time–frequency decomposition methods: empirical mode decomposition, discrete wavelet transforms, and wavelet packet decomposition with varying decomposition parameters. Various statistical and entropy-based features were computed from the decomposed coefficients. The statistical significance of these features was computed using Wilcoxon’s signed-rank (WSR) test for significance testing. The results of the WSR tests infer a significant change in many of these parameters after the consumption of coffee (caffeinated). Further, the analysis of the frequency bands of the decomposed coefficients reveals that most of the significant change was localized in the lower frequency band (<22.5 Hz). Herein, the performance of nine machine learning models is compared and a gradient-boosted tree classifier is proposed as the best model. The results suggest that the gradient-boosted tree (GBT) model that was developed using a db2 mother wavelet at level 2 decomposition shows the highest mean classification accuracy of 78%. The outcome of the current study will open up new possibilities in detecting the effects of drugs, various food products, and alcohol on cardiac functionality.
Ja tak, jeg vil gerne modtage nyhedsbrev, når der er noget nyt om kaffe og helbred.